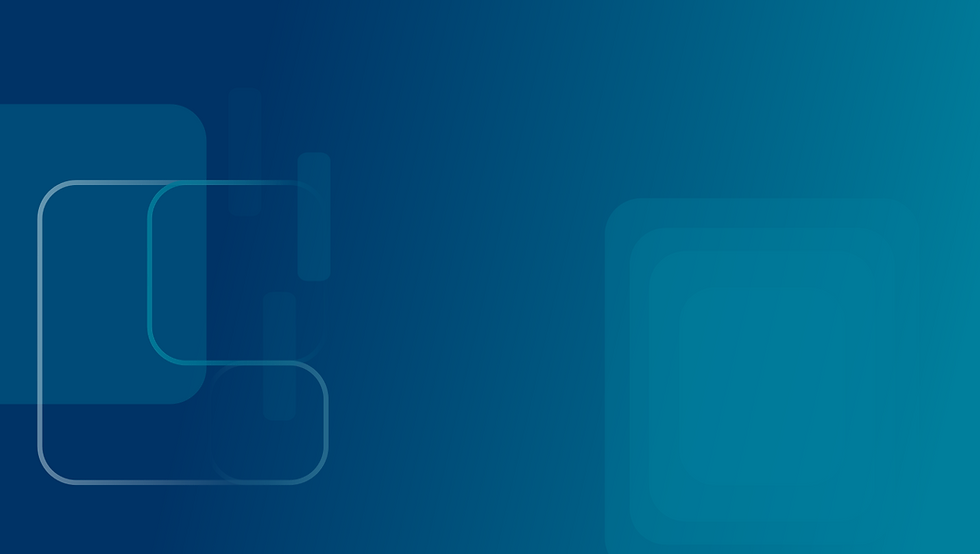
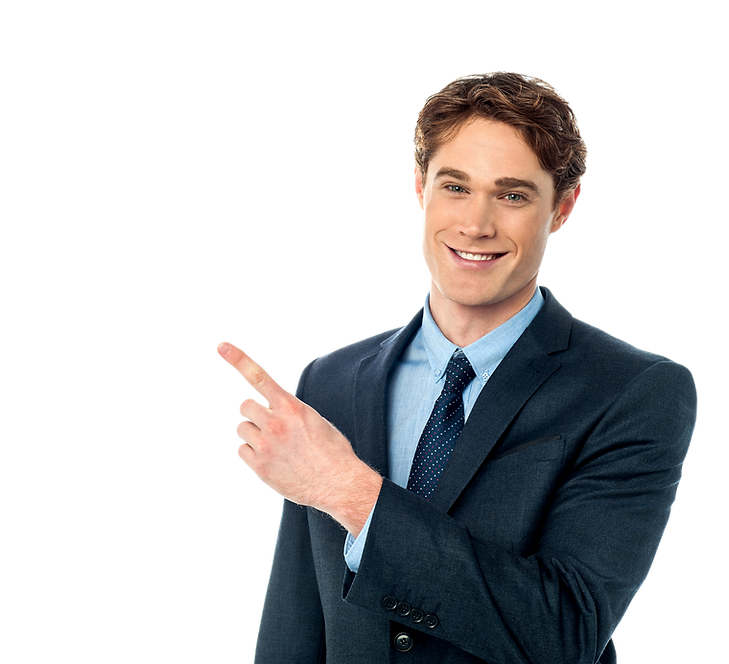.png)
Our Work
Derisk360 Project Overview
Data Quality Control for Batch and Streaming Data
Our endeavours in data quality control epitomize precision and excellence. Leveraging Apache Spark's formidable capabilities, we engineer a high-performance data processing framework designed to manage vast volumes of data with unwavering efficiency. Within the Hadoop ecosystem, we integrate Spark's advanced analytics functionalities, utilizing Spark SQL and Spark Streaming to ensure seamless processing of both batch and streaming data. Employing Python for scripting and automation, we orchestrate intricate data quality checks, including comprehensive validations for data completeness and integrity. Furthermore, by harnessing AI-driven anomaly detection algorithms, we enhance our quality control measures, guaranteeing the utmost accuracy and reliability in every data stream.

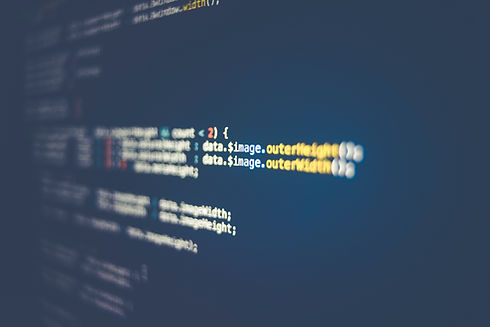
AWS Infrastructure Implementation for Banking Client
Embarking on AWS infrastructure projects, we uphold the highest standards of scalability, security, and reliability. Our meticulous approach involves deploying multi-tiered architectures on Amazon EC2, providing our banking clientele with resilient and adaptable computing resources. Leveraging Amazon RDS and DynamoDB, we address diverse data storage requirements, seamlessly integrating SQL and NoSQL database services into our infrastructure. Through the utilization of AWS Lambda for serverless computing and comprehensive security frameworks incorporating AWS IAM, Amazon Cognito, and AWS Shield, we fortify our infrastructure against potential threats, ensuring uninterrupted operations with unwavering vigilance. Moreover, by integrating AI-driven anomaly detection and prediction models, we continuously monitor and optimize our infrastructure, pre-emptively identifying and mitigating risks to uphold the highest standards of performance and security.
Master Data Management for Leading Network Rail Provider
Derisk360 proudly announces its strategic partnership with Hitachi Vantara in support of a prestigious UK leading network rail provider's Master Data Management initiative. Together, we're spearheading a transformative endeavour aimed at analysing and optimizing the client's diverse system landscape. The primary objective is to establish a centralized repository capable of efficiently managing client data, stakeholders, and critical processes. Through meticulous planning and strategic implementation, this initiative will streamline data management practices, enhancing operational efficiency and driving tangible improvements in organizational effectiveness. This collaborative effort exemplifies our commitment to delivering innovative solutions that empower our clients to navigate the complexities of modern data management with confidence and agility.
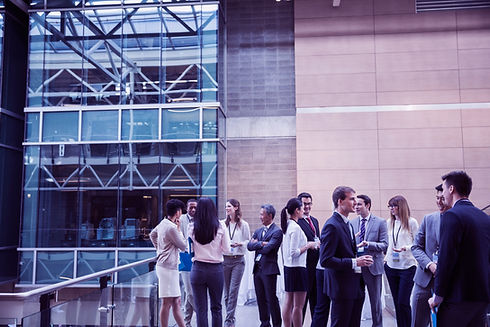
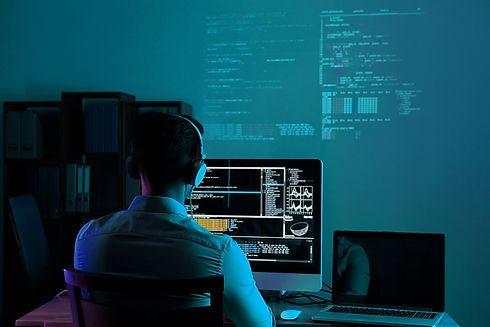
Large-Scale Data Migration Project
Within the realm of large-scale data migration projects, we exemplify proficiency and expertise in navigating complex data landscapes. Employing advanced AI algorithms, our approach is characterized by meticulous planning and meticulous execution, ensuring the seamless transition of data with minimal disruption. Through AI-powered data profiling and analysis, we meticulously assess the quality and structure of data prior to migration, facilitating efficient mapping and transformation processes. Furthermore, our utilization of natural language processing (NLP) algorithms enables the categorization and tagging of data elements, streamlining the migration process with unparalleled precision. Additionally, leveraging AI-driven data deduplication and cleansing techniques, we optimize data integrity and accuracy, ensuring a seamless and error-free migration experience from inception to completion.
AI-Powered Context-Based Q&A System Using RAG Framework
Our pursuit of AI-powered solutions transcends conventional boundaries, exemplifying innovation and sophistication. Harnessing the RAG framework, we develop a context-based Question and Answer system that embodies the pinnacle of artificial intelligence. Through the seamless integration of data into Chroma DB, a vector database optimized for efficiency, and the utilization of pre-trained models from libraries such as Hugging Face's Transformers for document embedding, we craft a system that is not only intelligent but also intuitive. Employing a microservices architecture built on Docker and Kubernetes, we ensure scalability and resilience, empowering our system to adapt and evolve with ease. Moreover, with AI at the helm, our system delivers unparalleled insights with unparalleled accuracy and clarity, revolutionizing the landscape of knowledge dissemination and retrieval.


Complex Data Pipelines Using Informatica & Stream Sets
Our proficiency in designing and implementing complex data pipelines epitomizes efficiency and ingenuity. Embracing Informatica PowerCenter and Stream Sets for high-volume data ingestion, transformation, and loading processes, our pipelines are meticulously engineered to optimize performance and scalability. Seamlessly integrated with Kafka for real-time data streaming and existing enterprise data warehouses and lakes, our pipelines facilitate end-to-end data flow management with unparalleled precision. Moreover, our commitment to security and compliance is unwavering, with encryption, data masking, and audit trails implemented to meet the stringent standards of the banking industry. Through AI-driven anomaly detection systems, we continuously monitor data flows, proactively identifying and mitigating potential risks to uphold the highest standards of data integrity and security.
Insurance Sector: Azure Data Management, RPA & AI-based UW Assistant
In our collaboration with a UK insurance company's specialty underwriting team, we embarked on an Azure-based data management overhaul, addressing challenges in managing internal/external, structured/unstructured datasets while ensuring UK-only data hosting. Concurrently, we developed a bespoke Product utilizing Microsoft RPA services tailored for Underwriting business needs. Challenges included inefficiencies in manual quote issuance, with each quote taking 20-30 minutes due to numerous manual steps, including cumbersome reference number creation within the Policy Administration System. Our Proof of Concept aimed to implement Robotic Process Automation (RPA) and an Underwriting Assistant, streamlining data processing, automating tasks, enhancing efficiency, automating quote generation, providing a user-friendly interface, and automating reference creation, all to optimize the underwriting process and improve overall productivity.
